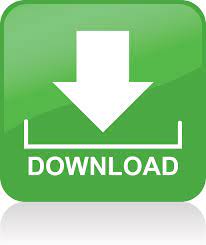

What we need here is a solutions that can overcome the drawbacks of put command and transfer the "streaming data" from data generators to centralized stores (especially HDFS) with less delay. Since the webservers generate data continuously, it is a very difficult task. If we use put command, the data is needed to be packaged and should be ready for the upload. Since the analysis made on older data is less accurate, we need to have a solution to transfer data in real time.

Using put command, we can transfer only one file at a time while the data generators generate data at a much higher rate. But, it suffers from the following drawbacks − We can use the put command of Hadoop to transfer data from these sources to HDFS. $ Hadoop fs –put /path of the required file /path in HDFS where to save the file You can insert data into Hadoop using the put command as shown below. Hadoop File System Shell provides commands to insert data into Hadoop and read from it. The main challenge in handling the log data is in moving these logs produced by multiple servers to the Hadoop environment. The traditional method of transferring data into the HDFS system is to use the put command.
#FLUME FOR WINDOWS SOFTWARE#
the application performance and locate various software and hardware failures.On harvesting such log data, we can get information about − For example, web servers list every request made to the server in the log files. Log file − In general, a log file is a file that lists events/actions that occur in an operating system. This data will be in the form of log files and events. Generally, most of the data that is to be analyzed will be produced by various data sources like applications servers, social networking sites, cloud servers, and enterprise servers. Hadoop is an open-source framework that allows to store and process Big Data in a distributed environment across clusters of computers using simple programming models. Big Data, when analyzed, gives valuable results. Using Flume, we can get the data from multiple servers immediately into Hadoop.Īlong with the log files, Flume is also used to import huge volumes of event data produced by social networking sites like Facebook and Twitter, and e-commerce websites like Amazon and Flipkart.įlume supports a large set of sources and destinations types.įlume supports multi-hop flows, fan-in fan-out flows, contextual routing, etc.īig Data, as we know, is a collection of large datasets that cannot be processed using traditional computing techniques. Some of the notable features of Flume are as follows −įlume ingests log data from multiple web servers into a centralized store (HDFS, HBase) efficiently. It guarantees reliable message delivery.įlume is reliable, fault tolerant, scalable, manageable, and customizable. The transactions in Flume are channel-based where two transactions (one sender and one receiver) are maintained for each message. When the rate of incoming data exceeds the rate at which data can be written to the destination, Flume acts as a mediator between data producers and the centralized stores and provides a steady flow of data between them.įlume provides the feature of contextual routing. Using Apache Flume we can store the data in to any of the centralized stores (HBase, HDFS). Here, Apache Flume comes to our rescue.įlume is used to move the log data generated by application servers into HDFS at a higher speed. To do so, they would need to move the available log data in to Hadoop for analysis. Applications of FlumeĪssume an e-commerce web application wants to analyze the customer behavior from a particular region. It is principally designed to copy streaming data (log data) from various web servers to HDFS. Apache Flume - Introduction What is Flume?Īpache Flume is a tool/service/data ingestion mechanism for collecting aggregating and transporting large amounts of streaming data such as log files, events (etc.) from various sources to a centralized data store.įlume is a highly reliable, distributed, and configurable tool.
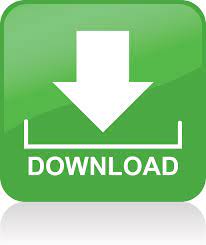